17/02/2025 - Blog
AI infrastructure of the future
“In traditional IT, you’d install an infrastructure stack that lasts you, say, five years. In AI, you’d only use it for two or three years before it’s hopelessly outdated. Think of Max Verstappen: if he used the exact same car for years, he’d dominate in the first year, lose a few races in the second, but not even touch the podium in the third.“
AI infrastructure of the future
Lorem ipsum
.png)
Testimonial
The course i attended gave me a great insight about data & ai. I would suggest it to everybody new and experienced people.
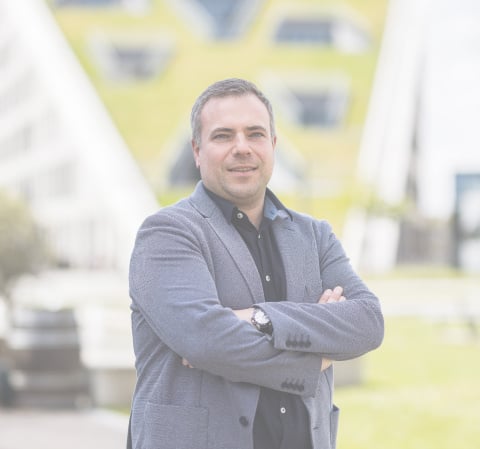
Jane Doe
UX Designer
Building the AI infrastructure of the future: an interview with MDCS.AI
With more organizations making big bets on artificial intelligence, the need for better data, more AI talent, and effective deployment strategies has only grown. The real challenge, though, lies under the surface: a robust AI infrastructure.
Few people are more aware of those needs that better than the tech savants at MDCS.AI. We sat down with Raymond Drielinger (CEO) and Niels van Rees (CCO), two experts with an obvious passion for AI infrastructure, to dig below the shiny surface of the AI industry.
As AI became the tech industry’s darling, almost every company wanted to start using AI. The need for better data infrastructure grew in parallel. The people at MDCS, an IT consulting firm at the time, picked up on that need early and decided to create an AI-focused arm called MDCS.AI, building on their existing track record in IT consulting and deep expertise in data centers. That bet paid off, as demand for data infrastructure soared and MDCS.AI became one of Nvidia’s so-called “elite partners”.
To start off, can you give us a non-technical idea of what we’re talking about when we’re talking about AI infrastructure?
Raymond: What do you need to run AI? First of all, you need GPUs - powerful processors that can handle huge workloads. What’s interesting about that is that these GPUs have to ingest massive amounts of data. So you’ll usually need a server system holding the GPUs, a data storage system that contains the data to be processed, and of course a link between those two systems – a network.
Niels: Many companies will try to run everything through the cloud, but that can get extremely costly over time, and it raises questions about how much control you have over your data. Some will want to move their AI Infrastructure locally or “on-prem”, or they might work in a hybrid system.
Raymond: The main problem with that is that most companies’ networks are simply not prepared to handle the data flows required by AI. They’ll be able to transfer, a certain number of gigabytes per second, while they might need something that’s 40 times faster. And sometimes, of course, we see startups working from a laptop. They’ll run their training program overnight and cross their fingers at breakfast, hoping the system hasn’t crashed completely.
Either way, you can’t fill up the gas tank of a Formula 1 car during a pit stop with a garden hose. You’d lose the race even before you started. So you need to be able to get enough data through your pipeline. That’s where we come in.
So IT expertise doesn’t necessarily transfer to AI expertise when it comes to infrastructure?
Niels: Not one-to one, at least. AI infrastructure is a very different ball game from what most IT experts are used to – it’s not just about servers and systems, but also about how you connect them. It’s a much more integrated approach that, in the end, is always a dense combination of software and hardware with a lot of moving parts.
It’s really like a puzzle. We often analyze how a specific workload and code runs on particular infrastructure, and sometimes we tinker with the levers and run something like an A/B test to check which combination works best.
Raymond: Our approach starts with our client’s needs. What are you trying to do? Where are the bottlenecks and pain points? Are you working in the most efficient way possible? It’s a process of mapping what’s needed below the surface to get everything to run perfectly. And if their use case requires particular expertise, we can loop people from the Nvidia network into the conversation.
Let’s zoom in on those bottlenecks. What are some of the most common challenges organizations face when scaling AI workloads?
Fragmentation can be a silent killer. Companies can go all-in on AI but fail to scale because they made the wrong choices in building their IT stack or their teams are all working on different things. Costs of running cloud workloads can also be a real issue. Moving your workload on-prem can get you out of that hitch but also requires a longer-term commitment.
Raymond: Some organizations have created “islands” where each team or group of researchers runs its own infrastructure. A single, shared platform would be much more efficient. Many people look at AI from a traditional IT perspective, where there’s a split between server, network, storage, and policy, and people work separately at each level. AI requires a much more integrated approach. There’s a lot of awareness-building to be done there, too, to accelerate performance.
So, is there a lot of change management involved, too?
Niels: In the end, everyone should get involved, because everyone’s a stakeholder. Are there any teams not using data today? AI is also much more cross-functional than just IT. But we don’t often see companies bringing all stakeholders to the table. And a lot of companies are rolling back IT outsourcing, simply because controlling your own IT is a competitive advantage.
The technical complexity of AI infrastructure might make it hard to articulate a coherent, organization-wide vision, then?
Raymond: Yes, but the pace of change makes it even harder. In traditional IT, you’d install an infrastructure stack that lasts you, say, five years. In AI, you’d only use it for two or three years before it’s hopelessly outdated. Think of Max Verstappen: if he used the exact same car for years, he’d dominate in the first year, lose a few races in the second, but not even touch the podium in the third.
Niels: Since AI is changing so quickly, what’s under the hood is changing just as quickly. There are other solutions than the traditional IT approach, though, like Life Cycle Management, where you ensure your AI infrastructure gets updated on a predetermined schedule.
Lastly, there’s been a lot of talk about the energy consumption of AI. How are you dealing with that?
Niels: We’re also talking to high-tech campuses to research how to build greener, more energy-efficient data centers. Looking critically at AI’s energy requirements and the way we can optimize resource consumption is vital for the future of AI as a whole – there’s no point in avoiding the issue. If AI is to be the technology of the future, we also have to build infrastructure for the future.
What does that mean for AI governance, risk, and compliance? Taking control over your AI infrastructure means you have a better overview of potential GRC issues. For instance, you can reduce your dependence on external (cloud) providers, which may reduce your overall risk exposure. You can also get a better overview of potential governance issues early on. Interested in finding out more? Get in touch with the experts at Umaniq.
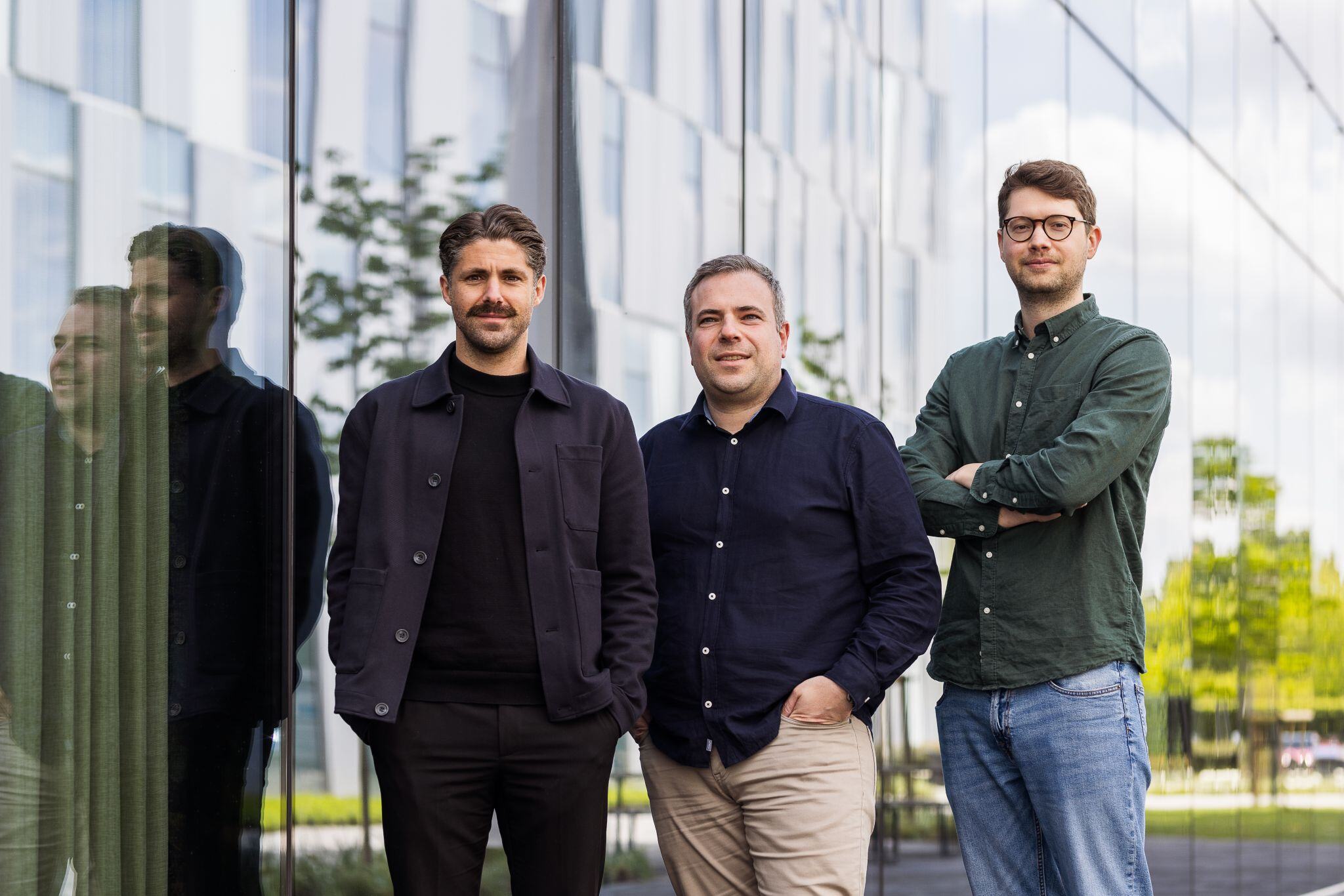
label text
Curious about the impact of the AI Act on your business?
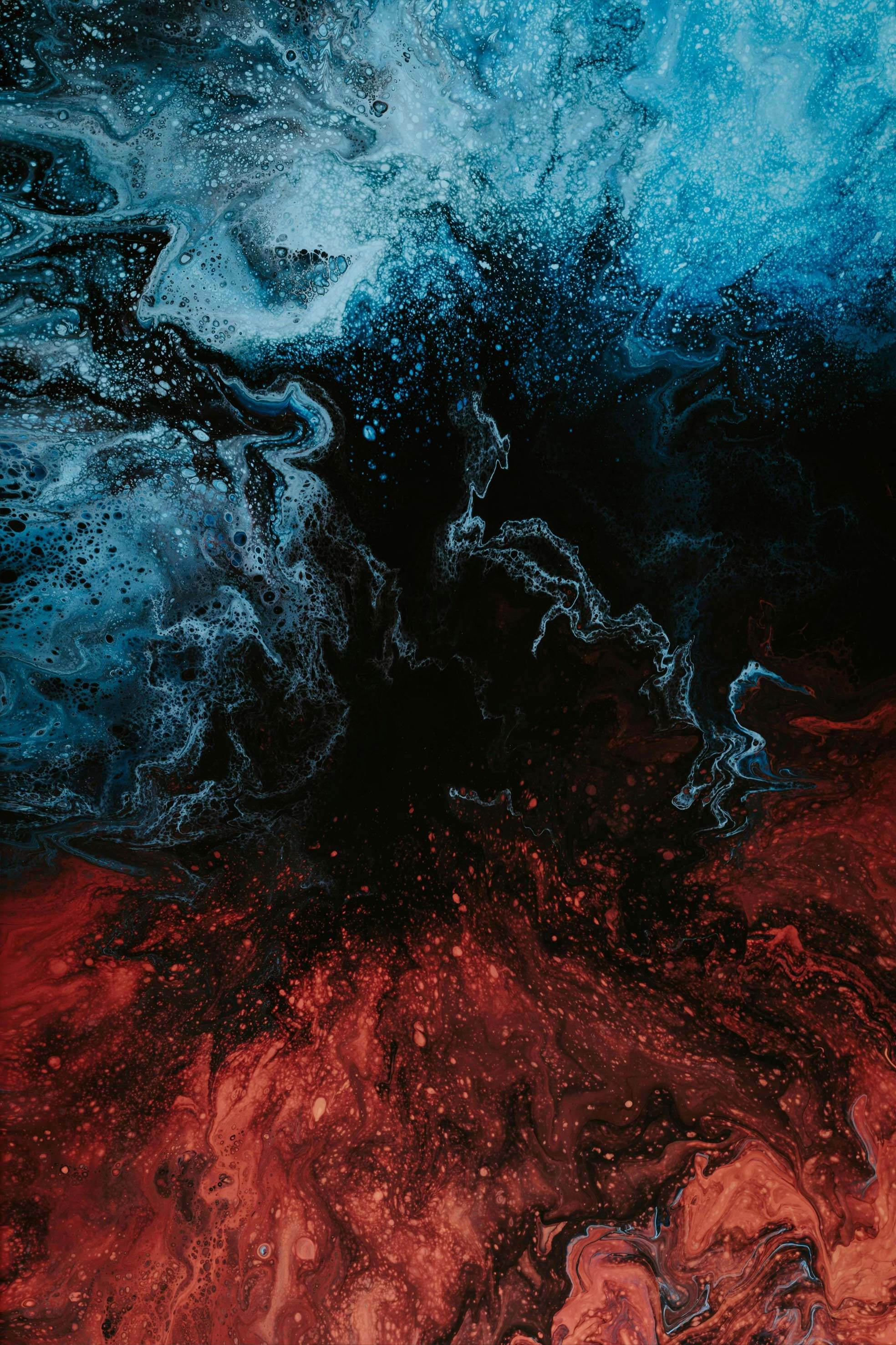
Jane Doe
UX designer